Sooner or later, all machines run to failure, but with a wide range of consequences. A sudden coffee maker breakdown can spoil your mood and morning. An unexpected malfunction in a power plant has the potential to leave thousands of people in total darkness for hours and cause a multimillion dollar loss.
The average cost of unplanned downtime in energy, manufacturing, transportation, and other industries runs at $250,000 per hour or $2 million per working day. To prevent expensive outages from happening and alleviate the damage caused by breakdowns, companies need an efficient maintenance policy. This article discusses available strategies, the benefits of the most advanced — predictive — approach, and resources required to implement it.
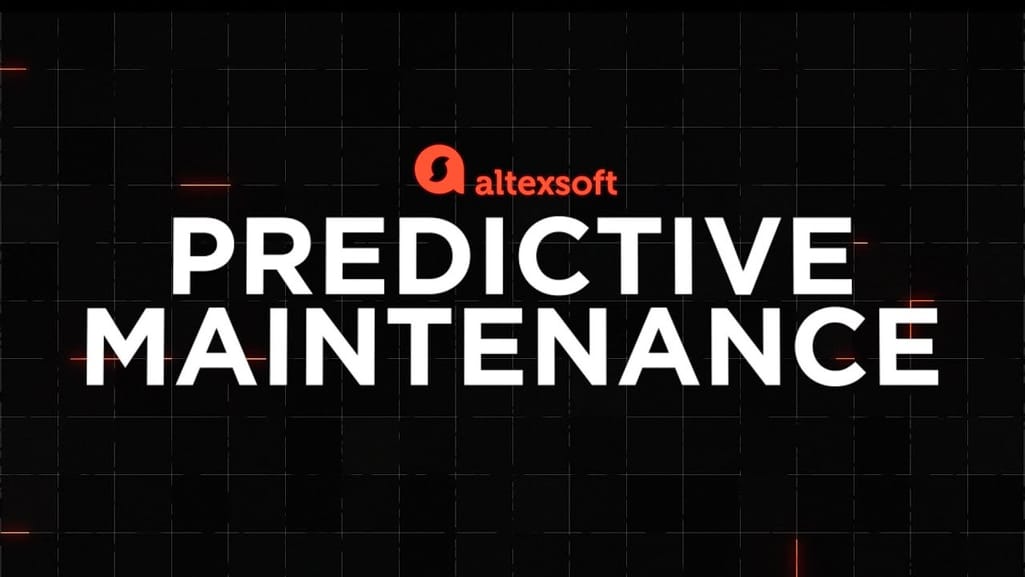
Predictive maintenance, explained
Maintenance policies: corrective vs preventive vs predictive strategy
There are three major types of maintenance policies a company can stick to: corrective, preventive, and predictive. Each option has its benefits and downsides, so let’s analyze them in more detail.
Cost allocation in different maintenance scenarios. Source: Tibbo Systems
Reactive maintenance: Fix the problem when it actually happens
Major pros: low maintenance cost, reduced amount of permanent staff, minimum planning required
Major cons: high repair cost, safety risks, potentially greater damage to machines
Reactive maintenance, also known as corrective, run-to-failure, or breakdown maintenance, means that the actions are taken when the equipment is already down. This approach saves time and money on planning and support services. It can take place in case of redundant, easy-to-repair, and non-critical assets. Say, light bulbs are replaced only after they burn out.
While corrective maintenance requires no initial costs, it turns out to be very expensive in the long run, considering overtime salaries, decreased useful life of assets, reputation damage, and safety risks. According to estimations by Marshall Institute, the reactive approach costs companies up to 5 times more than proactive types of maintenance.
Preventive maintenance: Fix everything on a schedule
Major pros: increased equipment efficiency and lifecycle, reduced likelihood of failures, money savings
Major cons: no way to exclude catastrophic failures, increased labor intensity and planned downtime, additional time on planning
Preventive maintenance triggers regular equipment inspections to mitigate degradations and reduce the likelihood of failures. Planned activities like lubrication or filter changes extend the useful life of assets and boost their efficiency. All this translates into money savings. Studies show that average savings from planned maintenance amount to 12-18 percent over reactive maintenance.
However, preventive measures can’t entirely exclude the possibility of catastrophic failures. Also, this practice involves planning and additional human resources. Often, the frequency of checks is higher or lower than needed to ensure reliability.
Predictive maintenance (PdM): Don’t fix what is not broken
Major pros: reduced maintenance time and cost, longer asset lifespan, reduced risks relating to safety, environment, and quality
Major cons: the need for organizational changes, large investments in hardware, software, expertise, and staff training
Predictive maintenance became possible with the arrival of Industry 4.0, the fourth industrial revolution driven by automation, machine learning, real-time data, and interconnectivity. Similar to preventive maintenance, PdM is a proactive approach to servicing of machines. The difference is that a company schedules activities based on constant condition monitoring. Once unhealthy trends are identified, damaged parts are repaired or replaced to avoid more costly failures.
The benefits PdM brings to businesses include lower costs on maintenance operations, prolonged equipment life, reduced downtime, a boost in production capacity, and enhanced safety. According to a report by Deloitte Insights, PdM promises
- a 20 to 50 percent reduction in time required to plan maintenance,
- a 10 to 20 percent increase in equipment uptime and availability, and
- a 5 to 10 percent reduction in overall maintenance cost.
But such improvements require significant investments in IT infrastructure and expertise — namely, in industrial IoT (IIOT) sensors, analytics software with machine learning capabilities, services of data scientists and IT specialists, and staff training. A company has to build an entire ecosystem that will support preventive activities. In the next sections, we’ll discuss when these efforts make sense and exactly what it takes to implement PdM.
Predictive maintenance use cases and factors of success
Which assets are worth applying PdM to? In the first place, this cost- and technology-intensive strategy justifies itself with high-value and critical equipment which must always be operational. Obviously, PdM is too expensive and not efficient for components that can be down for hours or even days without harming the production cycle. Everything in between needs additional deliberations to make the right choice.
Examples of companies successful at applying predictive maintenance.
Currently, most successful PdM is used in the following industrial sectors:
- manufacturing plants,
- power plants,
- railways,
- aviation,
- the oil & gas industry, and
- logistics & transportation (you can read about fleet maintenance and related technologies in a separate post).
Tasks you can solve with PdM
No matter the industry, when deciding upon implementation, you must clearly understand that PdM is only applicable to tasks predictable in nature. There are five major questions the PdM strategy can answer:
- What is the probability of a failure within a given time span?
- What is the remaining useful life (RUL) of the asset? In other words, how long will the machine run before it breaks?
- What is the likely cause of a given problem?
- What asset has the highest risk of breakdown?
- What activities will most efficiently solve the problem?
If your business is interested in attaining this kind of knowledge for improving maintenance procedures, PdM fits your requirements to a T.
Key factors enabling PdM
PdM strategy relies on several major pillars and just won’t work if any are absent or insufficient.
Data. The more data you have, the more informed decisions your maintenance staff makes. For generating accurate predictions, you have to collect and process real-time data from sensors, historical maintenance and failure records, equipment metadata, and even external information — like weather conditions.
Expertise. Besides training staff to understand PdM processes and work with new equipment, you need additional tech expertise. The efficiency of predictive maintenance largely depends on the following specialists:
- software and cloud engineers to integrate all parts of a predictive maintenance IT puzzle into one end-to-end solution and orchestrate their work;
- data experts to prepare data, choose, tune, and train machine-learning models, and interpret results; and
- reliability engineers to use results provided by data scientists for improvement of equipment efficiency and safety.
Considering the complexity of IT infrastructure required to run PdM activities, you may also need to involve an enterprise architect. This expert will evaluate your current systems, consult on available technologies, and help with major software and integration challenges.
IT infrastructure. PdM employs multiple hardware and software modules as well as cloud technologies. All components of the IT system are crucial for predictive maintenance activities, so we’ll investigate them in more detail.
Predictive maintenance IT ecosystem or technologies that drive PdM
Predictive maintenance involves constant data streaming from physical assets, analyzing real-time information against historical records, predicting outcomes, and thus mitigating or preventing potential breakdowns and downtimes. The smooth workflow is enabled by the orchestrated work of several systems and software solutions.
Main hardware and software components powering predictive maintenance.
IIoT system
IIoT devices or smart sensors that perform condition monitoring of equipment are the core of predictive maintenance. These built-in or external pieces of hardware capture physical parameters and translate them into digital signals.
Parameters used in predictive maintenance include but are not limited to
- vibration,
- current and voltage sensors,
- lubricant quality,
- liquid levels,
- temperature,
- pressure,
- sound levels and frequency, and
- chemical content.
Sensors stream signals to data storage through an IoT gateway, a physical device or software program that serves as a bridge between hardware and cloud facilities. It preprocesses and filters data from IIoT thus reducing its amount before feeding it to the data center. Besides, the gateway ensures connectivity, enhances security, and allows translation between different messaging protocols.
Computerized maintenance management system (CMMS)
A computerized maintenance management system is another important software behind PdM. It helps with controlling and analyzing all maintenance-related information — such as repair schedules, history of failures, spare parts usage, and maintenance activities as well as equipment specifications and technical requirements. Historical data accumulated over the years creates a solid foundation for accurate predictions.
Example of a CMMS dashboard with data on maintenance KPIs. Source: IAMTech
Central data storage
You need large and scalable storage to aggregate both real-time data from sensors and historical data from a CMMS.
It’s worth noting that very few companies have enough resources to keep information from sensors in on-premise data centers. Cloud solutions — namely, IoT and IIoT middleware platforms — are a better choice for collecting and storing large amounts of data. You can easily scale their capacity up and down, depending on the volume of data transferred and the number of sensors connected.
Analytical solution with machine learning capabilities
Simply collecting massive data sets from different sources is not enough. The predictive maintenance strategy requires powerful analytical tools relying on machine learning algorithms. Generating predictions with ML includes several phases.
- An analytical engine determines a normal health condition for the equipment based on historical data (learning phase).
- Data-based models are applied to constantly monitor the equipment’s health index.
- Once the system identifies signs of wear, it warns of problems so technicians can take action before the failure occurs.
Sometimes a predictive maintenance module comes as an integrated part of the CMMS, but it’s not always the case. Besides, this module can be hard to customize and lack machine learning capabilities, which results in a less accurate prognosis.
You may also use out-of-the-box applications that integrate with existing CMMSs as well as with IoT middleware platforms where IIoT data is stored. The leading analytical engines created especially for PdM tasks are:
- Senseye connects with a CMMS and builds machine learning models for prediction of Remaining Useful Life (RUL). It uses available historical data or learns machine behavior during 14 days before making predictions.
- Splunk, an industrial analytical tool already integrated with leading IoT platforms, gathers data from sensors, industrial control systems (SCADA), and CMMSs to perform predictive maintenance, leveraging statistical and machine learning capabilities.
- SentientScience is a digital platform that predicts the likelihood of failures in complex rotating equipment (turbines, railcars, rotorcraft).
In many cases, however, custom analytical solutions are needed to properly serve a specific industrial line or certain equipment.
Key industrial IoT platforms supporting PdM
Building an in-house PdM infrastructure from the ground up is costly, heavy on resources, and largely dependent on specific skills many industrial companies lack. But there’s a solution: industrial IoT platforms that give a solid foundation and necessary tools to unroll predictive maintenance activities. Varying in scope and the set of features offered, they usually provide companies the following capabilities and services:
- device management to connect thousands and hundreds of thousands of sensors and meters on one platform;
- support of industrial messaging protocols;
- software development environment, tools, and APIs to integrate with existing enterprise solutions;
- scalable data storage and a big data processing engine;
- analytics engines and machine learning as a service;
- Digital Twin technology — visualizations of the equipment’s condition in real-time; and
- ready-to-use asset management software and analytical engines tailored for PdM tasks.
Here’s a short review of some top industrial IoT platforms and their services.
Watson IoT by IBM
Reported advantages:
- backing by IBM, the global leader in artificial intelligence;
- a wide range of functionality;
- first-class IBM customer support in justifying, planning, and delivering custom applications.
Reported issues:
- complexity in terms of integration and development of an end-to-end solution;
- the high cost of services for small companies.
IBM Watson IoT platform is a cloud-hosted industrial solution with scalable data processing and storage services. It can handle tasks of varying complexity, from running the proof-of-concept to controlling fully-fledged production. The platform enables companies to register thousands of devices, apply AI-driven analytics to the data, and get insights into the performance and health of the equipment via visual dashboards.
IoT Watson device monitoring dashboard. Source: G2
Though the IoT platform provides you with a wide range of tools for monitoring sensors and running predictive maintenance, you still need software developers to use them and time to design applications. Companies that don’t have sufficient tech expertise or want to implement PdM as quickly as possible may benefit from the IBM Maximo Asset Performance Management suite of ready-to-use cloud-based applications. Among other modules, it includes:
- IBM Maximo Asset Monitor system for AI-driven management of physical assets on a single platform,
- Predictive Maintenance Insights application with five out-of-the-box predictive model templates, a library of analytics APIs to build custom models, and the ability to evaluate them using Watson Machine Learning.
Predix Platform by GE Digital
Reported advantages:
- vast industrial experience in aviation, manufacturing, oil and gas, and other sectors;
- a broad portfolio of software development tools for integration.
Reported issues:
- different development cycles and technologies used for building on-premises and cloud-based solutions which result in increased time and cost to support diverse use cases;
- the uncertainty caused by the growing debt of GE Digital parent — General Electric.
Predix Platform was created by GE Digital as middleware between the physical assets of multinational industrial conglomerate General Electric and its analytics platform. It allows for collecting data from heavy-duty machinery in centralized storage for performance diagnostics. In 2014, GE opened its IIoT platform for external companies, enabling them to manage data from sensors and build industrial applications powered by machine learning.
Predix Platform architecture. Source: GE Digital
The platform also offers a suite of Asset Performance Management (APM) software that includes a predictive maintenance component. Companies can use it to save time and money on software development services.
IoT Cloud Service by Oracle
Reported advantages:
- ease of integration with enterprise applications,
- fast implementation of ready-to-use business solutions,
- successful use cases of predictive maintenance across different types of industrial equipment, both in-plant and in-field.
Reported issues:
- reduced flexibility in comparison with self-directed IIoT platforms that provide an environment for custom software development;
- limited device management capabilities — Oracle solutions work with generic scenarios so you may need third-party solutions for managing some industry-specific devices.
Oracle IoT Cloud Service utilizes a broader Oracle Cloud Infrastructure and integrates with its middleware and enterprise software. Rather than a development environment, it offers business-ready solutions from Oracle’s portfolio like IoT Asset Monitoring Cloud with a predictive maintenance module.
The PdM tool leverages data streams from connected sensors, historical data, weather data, and other information collected in the IoT Cloud data lake. To derive valuable insights and deliver rich visualizations, it combines advanced analytics, machine learning algorithms, and Digital Twin technology.
Cumulocity IoT by Software AG
Reported advantages:
- support for over 150 devices and over 350 protocols to enable connectivity across a wide range of industrial sensors;
- fast no-coding implementation of industrial solutions,
- technological generality — the same APIs, models, and architecture are used for on-premise and cloud-based solutions.
Reported issues:
- problems with technical service and support for platform users,
- security concerns.
The main idea behind Cumulocity IoT is to let companies connect their devices and begin to work with data as quickly as possible. It comes with a large assortment of pre-built IIoT applications and optional add-ons like data management and predictive analytics tools. The promise is that an organization can run their pilot project within six weeks or less.
The platform also offers a software development kit (SDK) and support for microservices, enabling software engineers to create custom industrial solutions based on a microservices architecture. Thus, Cumulocity addresses the needs of both manufacturers who want to get quick solutions and providers of software development services for industries.
Steps to implement PdM: how to start small
With analytics technologies becoming more powerful and sensors getting cheaper, predictive maintenance is growing in popularity and usage. To understand what value PdM can bring to your business without painful financial cost, you can start with a proof of concept, applying the strategy to one machine or product line. The process will include several steps.
- Identify a critical piece of equipment.
- Define parameters to be monitored and install appropriate sensors.
- Engage an external team of data science experts to gather data and build machine learning models capable of extracting meaningful insights.
- Run the proof of concept for a couple of months to evaluate predictions against existing maintenance processes.
- Estimate annual savings.
If PdM implementation proves to reduce costs and enhance efficiency, scale it gradually to the entire enterprise, involving tech consultants to choose the right solutions, build custom software components, and assist with integrations.