In the continuously changing travel industry, data-driven insights are revolutionizing decision-making processes. Beyond its fundamental role in elevating customer experience, travel analytics has a valuable impact on revenue growth and cultivating a competitive edge.
This article explores the influence of analytics on marketing strategies, revenue management, guest personalization, and other aspects of the travel industry. We will examine key data sources, real-world use cases, and how to approach analytics in travel.
Internal vs. external travel data sources
At the core of effective travel analytics lies the fundamental requirement for diverse and robust data from internal and external sources.
Internal travel data sources
Internal data refers to the proprietary information generated and collected by an organization as a result of its day-to-day operations and interactions with clients. It provides a comprehensive overview of a company's performance, highlighting both successes and areas needing improvement.
By harnessing internal data sources, travel businesses can develop personalized services that cater to their customers' ever-evolving needs, as well as optimize their operations, enhance marketing strategies, and improve resource allocation, ultimately driving growth and profitability.
Company’s booking website and mobile app allow you to track and collect a wealth of data, from web traffic information to user behavioral metrics (session duration, navigation paths, etc.), geolocation, profiles, and feedback.
Internal systems — like a property management system (PMS) in hospitality, a passenger service system (PSS) used by airlines, or an OTA back office — are the backbone and the most relevant source of transaction details, booking histories, and inventory data for a travel business.
Learn more about the functions of internal systems with our dedicated articles about hotel property management systems and passenger service systems.
Customer relationship management (CRM) systems contain customer profiles with demographic information like age, gender, location, travel preferences, contact information, and communication history. They record and track customer and company interactions, spanning various communication channels like emails, chats, and phone calls. Loyalty program data can also be part of CRM records with information on membership tiers or rewards earned.
External travel data sources and providers
External data encompasses all types of information and datasets created outside a company and existing beyond its direct control, ownership, or management. Analyzing external data helps travel businesses stay competitive, adapt to shifting market conditions, and make well-informed choices regarding pricing, promotion, and overall strategies.
Other travel booking platforms are the main source of information on competitors' offerings. By monitoring and comparing them, you can identify popular services, destinations, and accommodations. This approach culminates in inventory optimization and competitive pricing strategies.
Social media and travel blogs contain valuable information about travelers' experiences, recommendations, preferences, and popular upcoming events. These online platforms include customer reviews and user comments, covering everything from current trends to destination-specific information.
Economic indicators cover a wide range of statistics that reflect the economic state of a region, country, or industry. They can be indicators of inflation, exchange rates, unemployment, and consumer spending. For the travel industry, this data is essential for understanding economic conditions that can impact travel patterns and consumer behavior.
Travel data providers offer a diverse range of ready-to-use data for hotels, travel agencies, and other stakeholders. For instance, a notable expert in this domain, OAG, has a suite of air-related datasets, including historical flight data spanning 20 years. Businesses selling vacation rentals can take advantage of data collection from AirDNA, Mashvisor, and others.
Read our article to find a more detailed exploration of short-term and vacation rental data.
Other external data sources that can be helpful when building travel analytics solutions are weather forecasts and reports, and information on events that take place in a particular location.
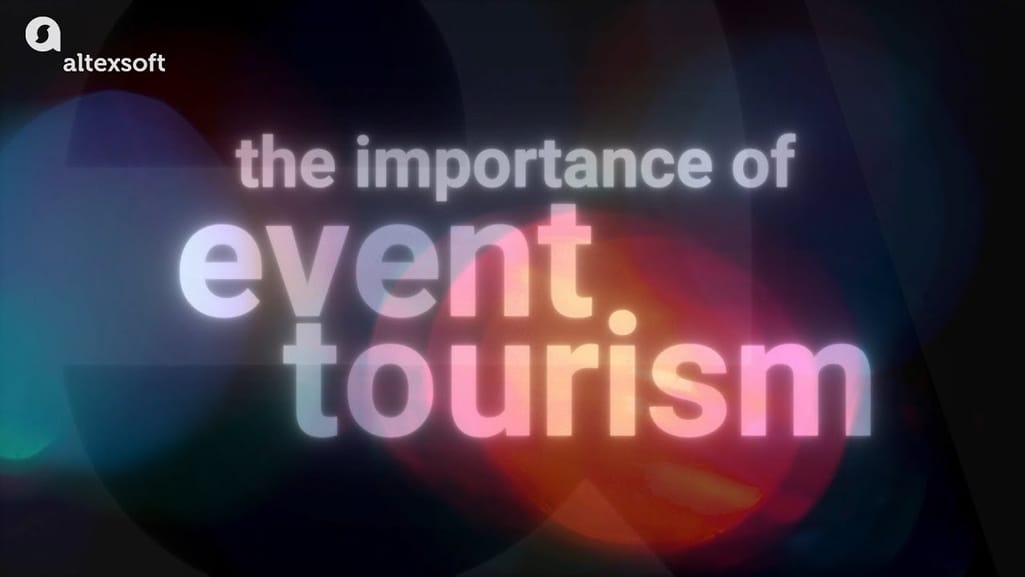
The importance of the events economy in the travel industry.
4 travel analytics use cases
The ability to apply analytical tools to vast amounts of information has become a game-changer for travel businesses. Here, we will look at 4 key use cases of travel analytics.
4 travel analytics use cases.
1. Revenue management
Revenue management is a set of practices for maximizing revenue by setting optimal prices based on demand. Many factors should be considered to find the right balance between rates and current seat availability, room occupancy levels, etc. That’s where modern analytical methods can help you out.
Demand forecasting with machine learning allows companies to spot the slightest shifts in consumer behavior and predict a probable future demand for a product or service. For instance, businesses can prepare for more customers when flight bookings intensify for a specific destination. They can adjust prices and create appealing travel packages.
Read our dedicated article to learn more about demand forecasting methods.
Price prediction empowers companies to maintain a competitive edge in the market by establishing optimal rates.
Learn about our experience in building machine learning models for flight price prediction and hotel price prediction.
Occupancy rate prediction is crucial for making data-based decisions on pricing strategy, planned maintenance, and staff scheduling. Accurate forecasts allow revenue managers to quickly identify periods of increased or decreased interest, for example, months in which rooms will be sold at a higher or lower price.
To gain further insights on this topic, take a look at our article that discusses occupancy rate prediction using machine learning.
Dynamic pricing is the process of setting the price of a product or service in response to current demand, market trends, and other factors. Demand forecasting, price prediction, and occupancy rate prediction can be part of a dynamic pricing approach, helping revenue managers maximize profits in ever-changing conditions.
2. Sentiment analysis
With the widespread adoption of social media, travelers often share their experiences and recommendations, providing businesses with information about customers’ perceptions and preferences. This data can be used to run sentiment analysis.
Sentiment analysis employs natural language processing (NLP), statistics, and machine learning to decipher emotions, opinions, and judgments in text by extracting subjective information. Also known as opinion mining, it categorizes sentiments as positive, negative, or neutral.
This type of text analysis can pinpoint areas for adjustment and assist in making improvements in service quality and customer experience, ultimately enhancing the brand's reputation.
Read our article about sentiment analysis in hotel reviews to learn how we generate instant feedback snapshots, enabling travelers to swiftly compare various options and make the optimal choice.
3. Personalizing customer experience
Over 70 percent of customers expect the companies they engage with to consider their unique needs and desires. By analyzing factors like age, goals, status, region, values, and interests, businesses can facilitate effective personalization strategies for the various consumer types. Recommender systems and trip planning tools are two popular examples of how analytics enable this process in travel.
Recommender systems use machine learning algorithms to make real-time personalized offers that meet the needs of a particular traveler. They can also aid in sustainable travel by suggesting ways to reduce carbon emissions and mitigate over-tourism, offering places with fewer visitors.
For a deeper understanding, read our article that covers recommender systems or watch our video about the main types of recommender systems.
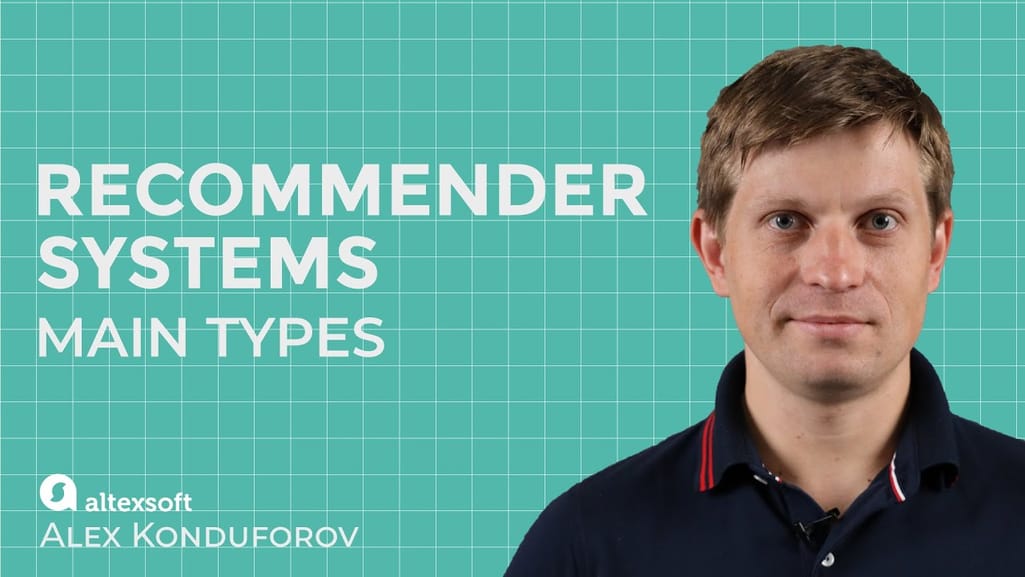
Main types of recommender systems.
Trip planning is another example of how analytics drive personalization in travel. It can be viewed as an advanced recommender system that generates an itinerary and recommends the best order of places to visit based on a traveler’s preferences, behavior, and other data. While some platforms go no further than proposing accommodations or ticket booking, more advanced services offer comprehensive trip arrangements, saving both time and money.
4. Risk management
In the travel industry, challenges can arise from various unexpected events. It’s important to surveil such situations and spot potential disruptions.
Fraud detection driven by analytics helps pinpoint subtle and hidden events in user behavior that can signal possible risks, such as fake bookings and chargebacks. To counter these risks, besides analytical tools, implement travel fraud prevention measures, including additional verification steps and raising the company staff's awareness of the fraud risk.
Read our article on ML-assisted fraud detection or watch this video:
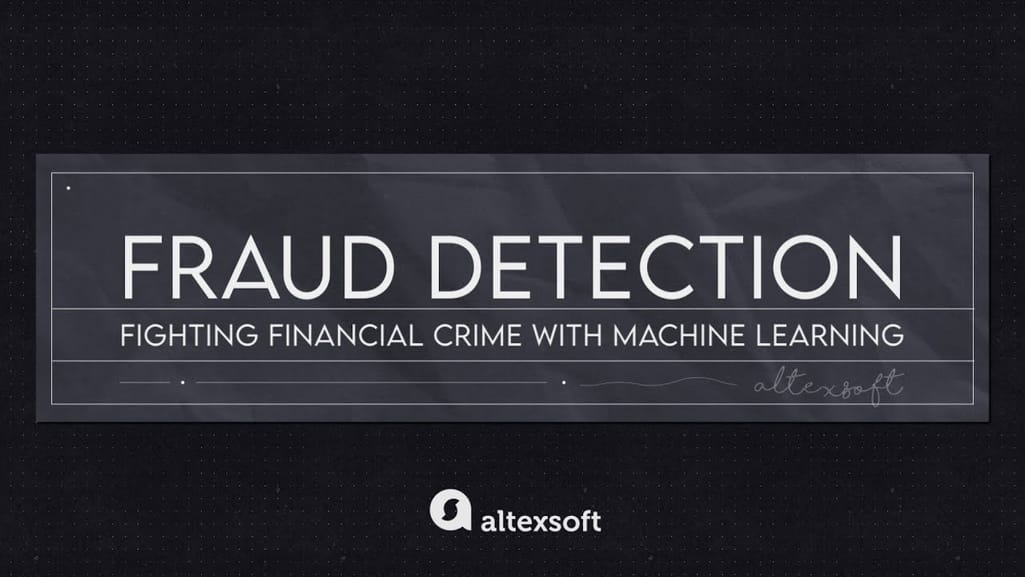
Fraud detection: Fighting financial crime with machine learning.
Guest screening is another application of travel analytics, involving the processing of guest information, booking details, and historical behavior patterns. In this examination, each reservation is assigned a risk score — a quantitative representation of the potential risk associated with that particular reservation. It takes into account factors such as the likelihood of property damage, the risk of non-payment, and the potential for illegal activities.
Guest screening equips hoteliers with the insights needed to make informed decisions regarding reservation acceptance. Identifying potential issues before guests arrive enables you to create a secure environment and mitigate risks that can impact the property's safety, reputation, and financial stability.
Flight arrival and departure time prediction is a powerful method for mitigating delay scenarios. Machine learning algorithms consider various factors like weather, arrival gates, aircraft type, and more, enabling airlines and ground handlers to better allocate gates, swap aircraft or crews on particular flights as needed, and thus minimize tarmac wait times.
Predictive maintenance utilizes machine learning to process data from IoT sensors and detect early signs of equipment failures. Due to this analytical method, airlines, car rental companies, and other businesses can address small problems before they develop into costly damages. For example, in aviation, predictive maintenance reduces the need to pull the aircraft out of service, helps optimize repair schedules, prevents unexpected faults, and insures against flight delays.
Real-life examples of successful travel analytics implementation
Here, we're sharing real-life examples of notable brands leveraging analytics to achieve impressive results in customer engagement, cost reduction, and operational efficiency.
Marriott: Bringing more personalization to the loyalty program
Marriott International, headquartered in Bethesda, Maryland, is a renowned American multinational company that operates, franchises, and licenses a diverse portfolio of lodging options, including hotels, residential properties, and timeshares. Marriott began as an A&W Root Beer franchise in Washington, DC, in 1927. Thirty years later, the company expanded into the lodging industry, marking a significant milestone.
To drive analytics, Mariotte partnered with IBM. After migrating to a modern data stack, Marriott deployed Db2 Warehouse on Cloud, which allowed IBM data scientists to map comprehensive customer data across all Marriott brands and build a travel experience platform that now powers Marriott Bonvoy, the industry's largest loyalty program with over 140 million members. Each time customers redeem their loyalty points, the platform gains more data to better understand those travelers and market to them in a more personalized way.
American Airlines: Optimizing operations and reducing fuel consumption
American Airlines, founded almost 100 years ago, has a deep-rooted legacy of pioneering achievements, launching the first major carrier loyalty program, and introducing airport lounges. Headquartered in Fort Worth, Texas, it is the largest airline globally in terms of scheduled passengers carried and revenue passenger miles, a metric used to measure the total miles traveled by paying passengers.
After transitioning its data platform to Microsoft Azure, American Airlines got a specific focus on optimizing operational efficiency. At the heart of its analytical efforts is an intelligent gating program that leverages real-time data on routes and runways and automatically assigns the nearest available gate to arriving aircraft. This enabled significant reductions in taxi time and fuel consumption, saving up to 10 hours of taxi time per day and thousands of gallons of jet fuel per year.
American Airlines also developed the Hub Efficiency Analytics Tool (HEAT), an ML-driven program implemented to minimize the impact of weather-related flight delays. It analyzes various data points, including weather conditions, customer connections, air traffic, and gate availability. HEAT's proficiency in dynamically adjusting departure and arrival times has decreased flight cancellations during adverse weather conditions.
All told, American Airlines' strategic embrace of AI, machine learning, and data analytics has led to notable improvements in operational efficiency, customer experience, and cost reductions.
Hilton: Personalization with data-driven smart suggestions
Hilton Worldwide Holdings Inc. is a renowned American multinational hospitality company headquartered in McLean, Virginia. From its inception in 1919, Hilton's goal was to operate the finest hotel in Texas. Commitment to excellence and innovation has positioned it as one of the world's most respected brands that has grown to operate in over 100 countries.
Recognizing the growing importance of data in hospitality, the company is constantly investing in analytics to stay ahead in an ever-evolving market. For example, Hilton implemented in-page data capture technology by Celebrus, to accurately capture the smallest details of customer behavior on hotel sites. It allows for a better understanding of visitors and enhances their experience.
Hilton also benefits from OnQ CRM, a proprietary system developed to handle large amounts of diverse customer data collected through various communication channels. With predictive analytics, the company can use these large volumes of data to forecast customer retention and better meet guest demands. Incorporating advanced data analytics and innovative technologies, Hilton has not only solidified its position as one of the global hospitality leaders but has also demonstrated its commitment to an exceptional guest experience.
Agoda: Enhancing user journey with OpenText Analytics
Agoda, founded in 2005, has revolutionized travel by simplifying the search and booking process. This Singapore-based OTA operates globally and is considered one of Asia's leading travel-tech companies. With millions of registered customers and over 6,000 employees across 31 markets, Agoda offers access to over 2 million properties worldwide and a range of travel-related services.
Every day, the company's website serves millions of customers, which makes a frictionless booking journey and overall usability a major competitive factor. “Customers will stick with us if we provide high-quality products and a streamlined experience, and accommodation owners will offer optimized pricing if we give them the best marketplace," Idan Zalzberg, VP Data at Agoda, explains.
To stay ahead of rivals, Agoda employed Vertica Analytics Platform (now owned by OpenText.) It enabled the OTA to analyze complex user behavior patterns in seconds and constantly improve the website based on insights from large volumes of data.
How to approach travel data analytics
The process of unlocking valuable insights from data involves the following key steps.
Assemble a data science team. Building or hiring a skilled data science team with expertise in the travel domain is a fundamental step to take before the process even begins. Pay attention to the collaboration and communication skills of the team members since they must interact a lot with other stakeholders and be on the same page with them.
Formulate key questions. Clearly define the problem your organization is going to solve with the help of analytics. Establish measurable goals and KPIs to gauge the success of your efforts. Articulating the anticipated results is critical for ensuring that all stakeholders have a shared understanding of the project's purpose and direction.
Define data sources and collection methods. The next crucial phase is to gather the necessary data for analysis. The choice of data sources and collection methods should align with business objectives to ensure that the analysis is based on accurate and relevant information. Define the timeframe for data collection and create a clear plan with information on team assignments, relevant data sources, and key requirements.
Choose analytical tools. Depending on the nature of the data and your business objectives, a variety of techniques may be employed, including machine learning, deep learning, natural language processing (NLP), and computer vision. Discuss the most appropriate methods with your data science team.
Communicate and visualize the results. This step ensures that stakeholders understand and can use the analytics results to make informed decisions. Engage with stakeholders in a way that resonates with their level of expertise and interest, maintaining clarity and transparency. To enhance effective storytelling, utilize BI tools like Power BI or Tableau, or Python libraries like Matplotlib and Seaborn.
Read our article on data visualization libraries and tools to learn more.
Consider implementing data governance. An effective data governance strategy ensures the accuracy, availability, and security of data, making it compliant with data regulations, such as the GDPR in the EU and CCPA in California. Data governance policies safeguard the company’s reputation and enhance both partner and customer trust.
These steps are crucial to fully leverage the power of data in your travel operations and ensure that your business initiatives are geared towards making informed, customer-centric decisions.